GitHub Repository
Overview
This project involves the development of an intelligent mobile robot that navigates autonomously within a known indoor environment. The system utilizes a pre-generated map for navigation and a line-tracking localization method (Suivi de ligne). Task planning and execution are based on the Block City (Manhattan) algorithm. The graphical user interface (GUI) for real-time monitoring and control is built using LabVIEW.
Implementation Details
-
Localization and Navigation
- The robot uses a line-tracking localization technique to follow predefined paths.
- Odometry and sensor data, including ultrasonic sensors, help with localization and obstacle avoidance.
-
Mapping
- The robot operates in a known environment with a pre-generated map using Cartesian coordinates.
- Sensors and algorithms continuously update the map during navigation to account for environmental changes.
-
Task Planning
- The Block City (Manhattan) algorithm is employed for calculating distances and optimal paths for task execution.
- Tasks include simple object manipulation like picking and placing objects within the environment.
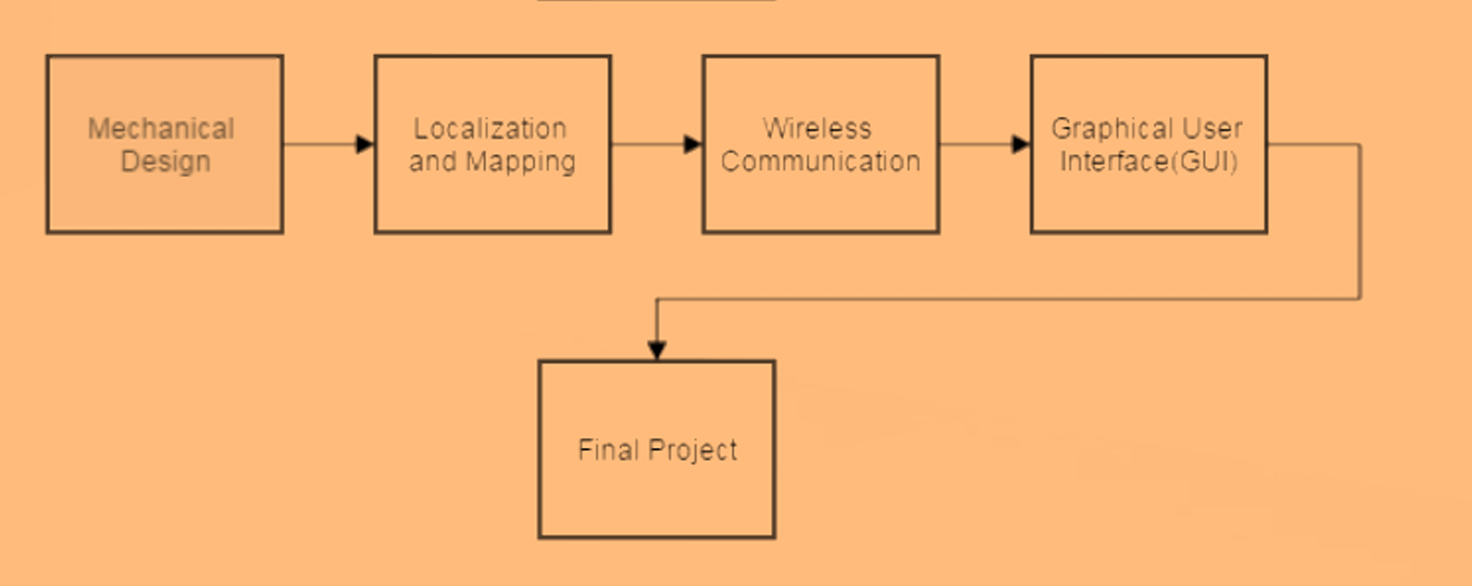
- User Interface
- LabVIEW is used to create a real-time GUI that displays the map, robot’s location, and task progress.
- The GUI allows for intuitive control and monitoring of the robot’s status.
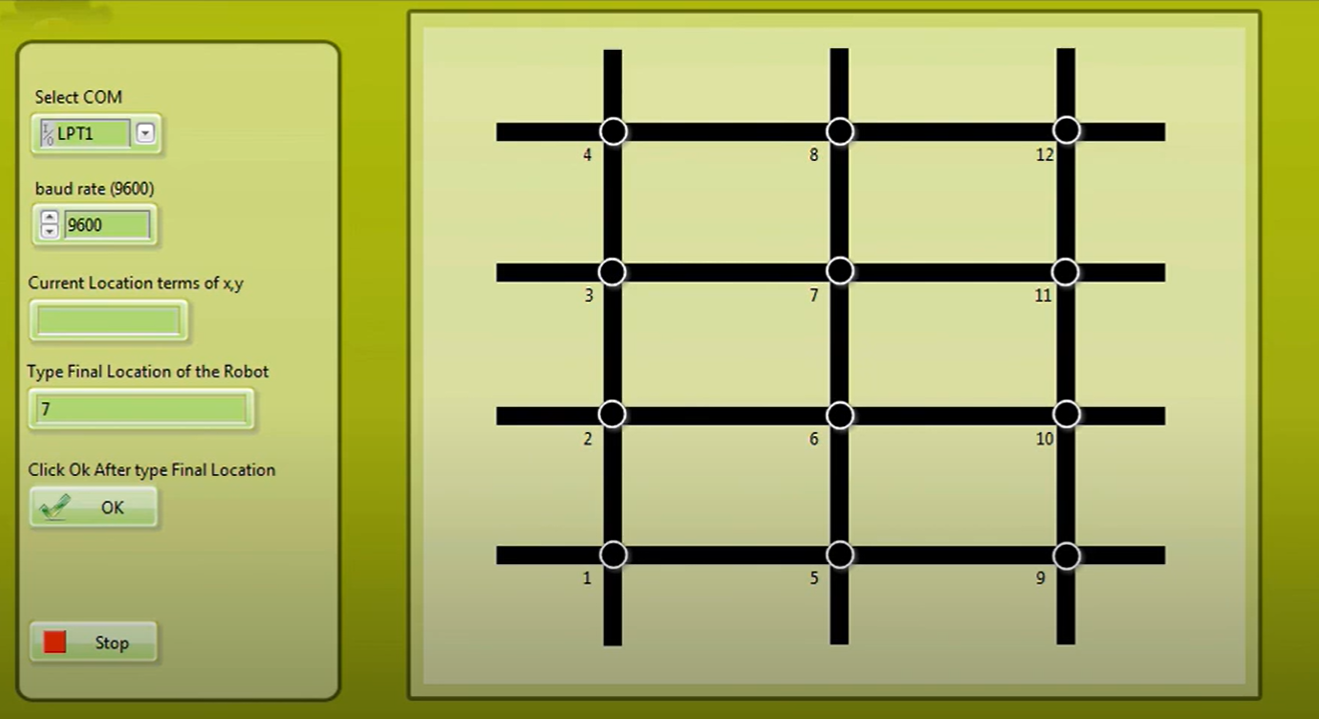
- Hardware
- The robot is built on an Arduino platform using motors and sensors to interact with the environment.
- It includes modules for communication via RF and is capable of receiving commands from external devices.
Technologies Used
- Arduino: Control and communication, sensor integration.
- LabVIEW: For building the graphical user interface (GUI).
- Block City (Manhattan) Algorithm: For task planning and navigation.
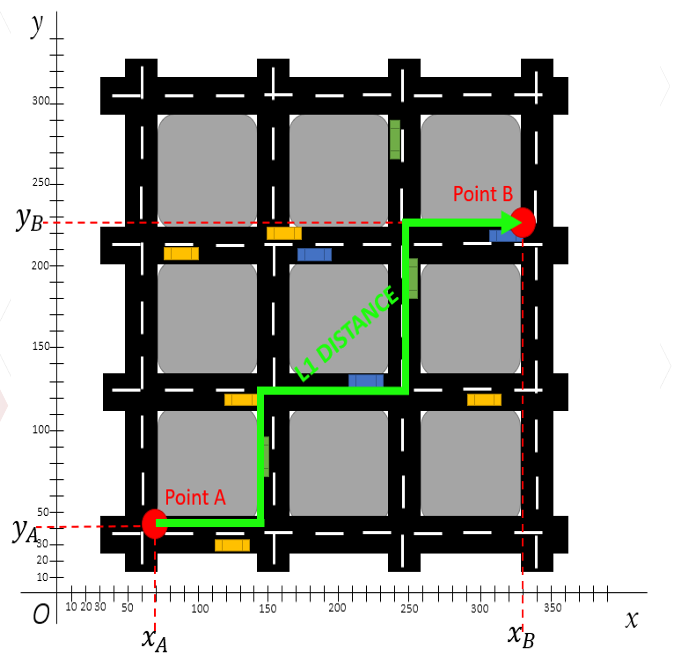
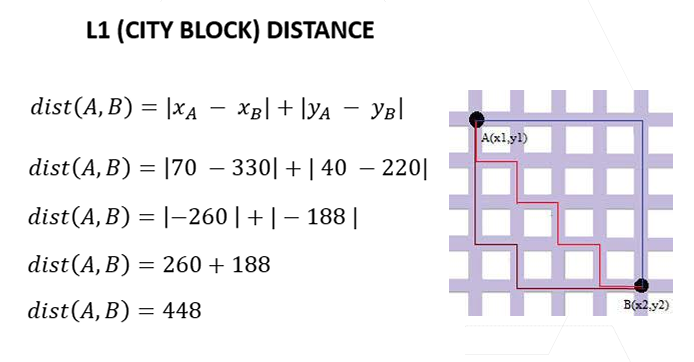
- Line-Tracking Localization: For position estimation in indoor environments.
- Ultrasonic Sensors: For obstacle detection and environment sensing.
Results & Findings
- The mobile robot demonstrated effective indoor navigation, successfully completing tasks such as picking and placing objects.
- The real-time GUI built using LabVIEW significantly enhanced the usability and monitoring of the robot’s activities.
- The robot’s localization method provided accurate navigation within the predefined map.
Future Improvements
- Advanced Localization: Implement SLAM (Simultaneous Localization and Mapping) for dynamic environments.
- Task Expansion: Integrate more complex tasks and improve task planning algorithms.
- Energy Efficiency: Implement power management techniques for better autonomy.
Contributors
- Imad-Eddine NACIRI
- Achraf Berriane
- Errouji Oussama